As the world’s population continues its relentless climb, optimizing food production has become a paramount challenge. Fortunately, the burgeoning field of artificial intelligence (AI) and machine learning (ML) is revolutionizing the agriculture and horticulture industries. Leveraging cutting-edge technologies, man-made intelligence is enhancing efficiency, yield, and sustainability for greenhouse growers, nurseries, and farms of all sizes through powerful data-driven insights.
From automated environmental controls to predictive analytics and autonomous robotics, AI/ML solutions are rapidly transforming how we cultivate crops and manage growth cycles. This blog post will explore the innovative applications of man-made intelligence in horticulture, illuminating a future where precision agriculture and technology converge to feed the world more intelligently.
AI-Powered Environmental Control Systems
At the core of precision horticulture lie intelligent environmental control systems driven by artificial intelligence. By continuously monitoring and analyzing real-time data on light intensity, humidity, temperature, soil conditions, and other key variables, these systems can automatically make granular adjustments to create the optimal predictive growing conditions.
Take the example of modern smart greenhouses that utilize AI-powered climate controls. An intricate network of sensors and cameras capture comprehensive data, which is then fed into machine learning models. These models identify complex patterns and relationships, enabling the greenhouse to self-regulate heating, ventilation, irrigation, shading systems, and more with minute accuracy.
The benefits of such advanced environmental control are multi-faceted:
- Reduced resource usage: AI systems can optimize inputs like water and energy, minimizing waste and costs.
- Increased productivity: By maintaining ideal conditions, growth rates and yields are maximized across crop cycles.
- Consistent quality: Eliminating environmental variability results in more uniform, higher-grade products.
As a case study, Revol Greens, a greenhouse lettuce grower, implemented an AI-controlled environment system which boosted productivity by 8% while slashing energy costs by 5%. Their AI tech partner, Irvine, stated it “creates a perfectly tailored environment for each stage of the growing cycle.”
Machine Learning for Crop Management
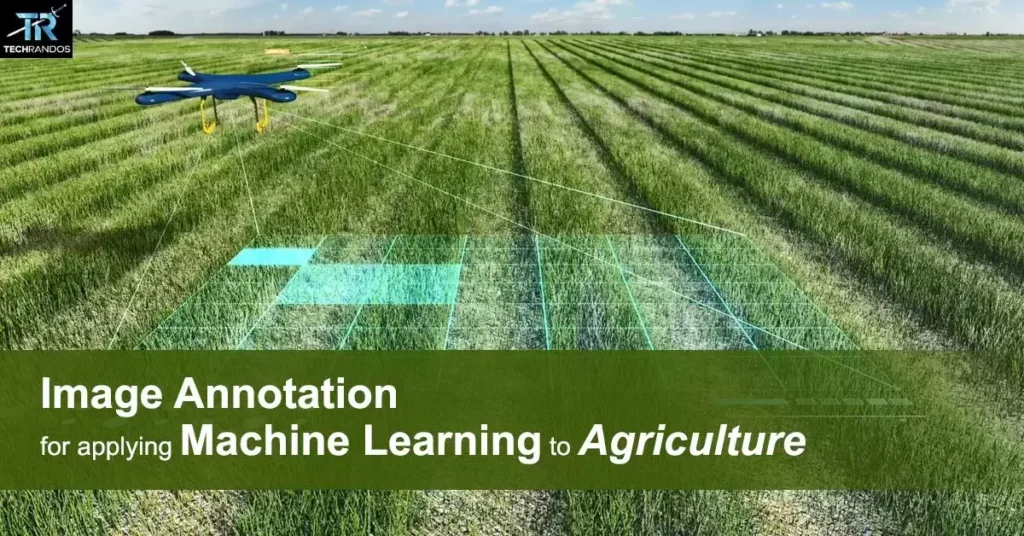
Another crucial application of man-made intelligence in horticulture lies in leveraging machine learning algorithms to analyze a wealth of data on pests, diseases, growth patterns, climate factors and more. By uncovering deep insights from this big data, AI/ML solutions can drive predictive analytics that optimize every step of the crop management process.
For instance, ML models can forecast ideal planting times, streamline soil preparation through precise nutrient/amendment recommendations, schedule irrigation with laser-focused efficiency, and detect pathogens or nutrient deficiencies at the earliest possible stage through visual image recognition of subtle symptoms.
Benefit | Use Case |
Improve Yield Forecasting | Analyze historical data plus real-time conditions to predict crop yield with high accuracy weeks/months in advance. Enables better planning and logistics. |
Reduce Crop Losses | Early detection of pests/diseases allows for rapid targeted response, preventing widespread damage and losses. |
Optimize Inputs | Precision application of fertilizers, pesticides, water, etc. tailored to specific crop needs boosts efficiency and eco-sustainability. |
A notable example is the work of researchers from Penn State, who developed an AI-based system that can identify crop diseases from smartphone photos with 98% accuracy. Their platform “AI Planters” decodes patterns invisible to the human eye, making it a powerful diagnostic tool for growers.
Recommended Post: Unleashing the Power of Simulated Intelligence for Cutting-Edge Computer Vision
Autonomous Robotics in the Growth Cycle
While environmental controls and crop analytics have been revolutionized by AI/ML, another key area being upended is labor optimization through autonomous robotics. Combining advanced computer vision, mechatronics, and intelligent motion control, modern robotic solutions can take over many of the repetitive, labor-intensive tasks throughout the horticultural growth cycle.
From delicate seeding and de-leafing, to pruning, thinning, picking, and harvesting – robotic systems can perform these complex actions with astonishing precision and non-stop productivity. In fact, next-generation robots with enhanced dexterity and AI guidance can mimic the nuanced hand movements and decision-making of expert human workers.
A prime example of such innovation is the UV robotic systems created by Ultramen for integrated pest management (IPM). Using computer vision and autonomous navigation, these intelligent robots can roam greenhouse aisles 24/7, continuously scanning plants and administering targeted UV-C light “baths” to sterilize pests while avoiding harm to crops or workers.
The benefits of deploying autonomous robotics extend far beyond just labor savings:
- Greater productivity: Not limited by human constraints, robotic systems can operate around the clock.
- Reduced costs: Optimized labor spending with enhanced precision and minimal crop losses.
- Labor shortage solution: Offsets the growing deficit of available human workers.
- Increased safety/quality control: Eliminates human error and ensures consistent processes.
While upfront investments can be significant, the productivity gains, cost reductions, and scalability offered by horticultural robotics with AI/ML integration make them economically compelling long-term solutions.
Challenges and Limitations
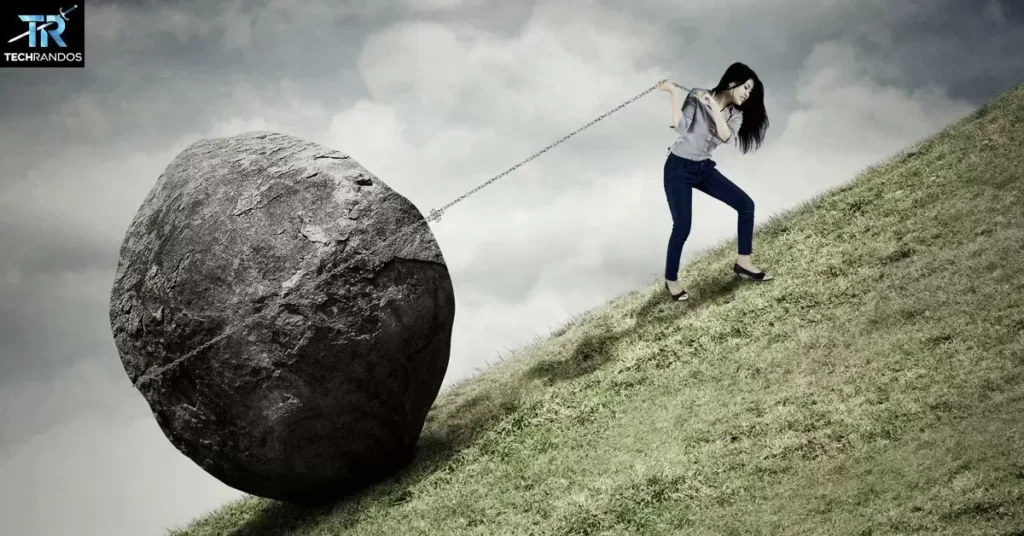
Despite the transformative potential of man-made intelligence in horticulture, there remain some critical challenges and limitations that must be addressed:
Data Quality/Availability Barriers: AI/ML systems are only as effective as the data they’re trained on. Inconsistent, incomplete or biased datasets can hamper accuracy. There’s also a lack of robust historical agricultural data in many regions.
Localized Variability: A key issue is accounting for hyperlocal variability in micro-climates, soil compositions, pest/pathogen prevalence and other geographic factors that can trip up AI models.
Integration Obstacles: For farms/operations with a mix of new and legacy equipment, integrating disparate systems and data streams into a unified AI workflow can prove complex and costly.
High Start-Up Costs: Implementing cutting-edge AI systems, robotics, advanced sensors, etc. often requires significant capital expenditure – creating affordability barriers for smaller operations.
Workforce Disruption: While automation can offset labor shortages, it also inevitably leads to concerns around personnel displacement and workforce retraining needs.
Moving forward, collective efforts from technology providers, agricultural organizations, researchers, and policy makers will be vital to mitigating these roadblocks in a responsible manner.
The Future of AI in Horticulture
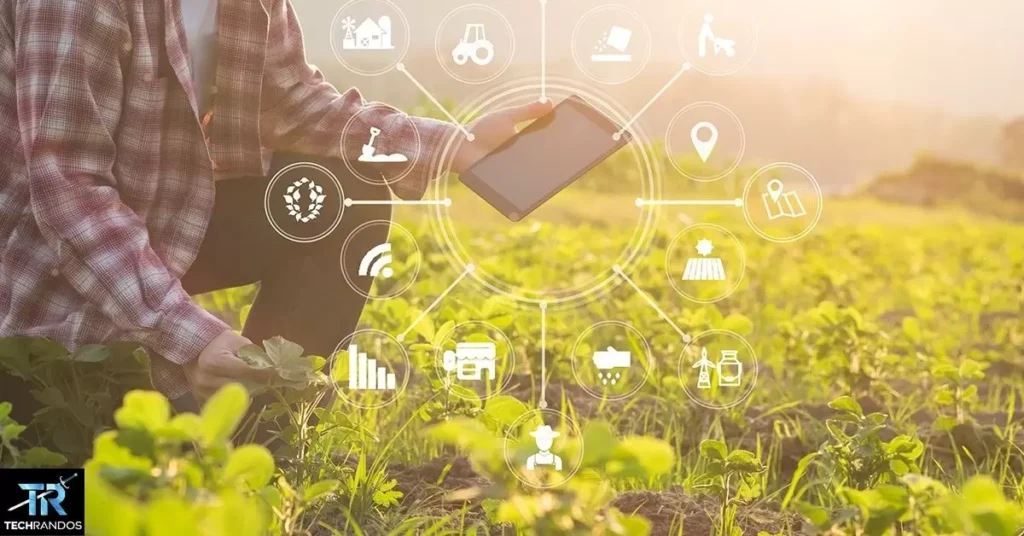
Even as AI rapidly enhances modern horticulture, the future promises even more incredible developments and opportunities driven by man-made intelligence:
Emerging Technologies
- Hyperspectral Imaging: By detecting hundreds of spectral bands, this advanced variant of image analysis can reveal insights about crops impossible with standard RGB cameras.
- Digital Twinning: Creating virtual replicas of physical grow environments powered by real-time sensor data enables extensive AI-driven scenario modeling.
- Edge AI: Moving AI computation directly onto farm machinery, vehicles, and robotic devices will unlock new capabilities without cloud connectivity.
Sustainability Advantages: A core advantage of AI/ML is optimizing inputs like water, fertilizers, pesticides – indirectly boosting eco-sustainability. Future solutions may even be able to autonomously compensate for conditions like drought.
Autonomous Grow Operations: Integrated AI platforms combining environmental controls, crop analytics, robotic systems and more could theoretically manage entire grow cycles with very limited human oversight needed.
Urban Agriculture Enablement: By streamlining processes and maximizing space/yield, AI may make urban vertical farming models and other non-traditional growing methods increasingly viable and scalable.
Frequently Asked Questions
Q: What is man-made intelligence in horticulture?
Man-made intelligence refers to the use of artificial intelligence (AI), machine learning, robotics, and other advanced technologies to enhance operations and productivity in the horticulture industry.
Q: How does AI help with environmental control in greenhouses?
AI-powered systems can monitor and analyze data on light, humidity, temperature, soil conditions, and more. They then automatically adjust heating, irrigation, shading and other controls to create the optimal growing environment.
Q: What are some benefits of using machine learning for crop management?
Machine learning can forecast ideal planting times, optimize soil prep and irrigation, detect pests/diseases early through image recognition, reduce crop losses, and improve overall yield predictions.
Q: How are robots used in horticulture growth cycles?
Autonomous robotic systems with AI guidance can take over labor-intensive tasks like seeding, pruning, de-leafing, and harvesting crops with precision and 24/7 productivity, helping address labor shortages.
Q: What are some challenges with adopting AI in horticulture?
Key challenges include data quality/availability issues, accounting for hyperlocal variability, integration complexities with legacy equipment, high startup costs, and workforce retraining needs.
Q: What emerging AI technologies may further impact horticulture?
Hyperspectral imaging, digital twinning of grow environments, and edge AI computing could unlock new capabilities. AI may also enable fully autonomous grow operations.
Q: How can AI help make horticulture more sustainable?
By optimizing inputs like water, fertilizers and pesticides, AI solutions boost eco-sustainability indirectly. Future AI may even autonomously compensate for climate impacts like drought.
Conclusion
There’s no doubt that man-made intelligence in horticulture is ushering in a pivotal transformative era. By harnessing the power of AI, machine learning, robotics and big data, growers can transcend conventional limitations to achieve unprecedented levels of precision, productivity and sustainability.
From automated greenhouses that self-optimize every environmental factor, to AI diagnostics safeguarding crops, all the way to fleets of autonomous robotic farmhands – the future of agriculture is becoming ever-more intelligent, efficient and scalable in the face of monumental food supply challenges.
However, as revolutionary as these AI/ML technologies prove, their successful real-world deployment hinges on a strategic, holistic approach that accounts for data readiness, integration complexities, economic feasibility and responsible human workforce transition.