Man-made intelligence in production networks is revolutionizing the industry. This innovative technology streamlines processes, boosts efficiency, and enhances decision-making. Companies leveraging AI see improved supply chain management and reduced operational costs. Discover how AI is transforming production networks into agile, intelligent systems. Stay ahead with insights on this game-changing advancement.
What is Man-made Intelligence in Production Networks?
Man-made intelligence refers back to the application of AI technology inside production networks permitting machines to perceive, analyze, cause, and make information-driven decisions. In an commercial context this includes leveraging superior algorithms and large quantities of data to streamline procedures beautify first-class manage and pressure operational efficiencies.
Some common use cases of man-made intelligence in production networks include:
- Predictive Maintenance: AI-powered systems can analyze real-time sensor data from machinery and equipment to predict potential failures and schedule maintenance proactively, minimizing costly downtime.
- Defect Detection: Computer vision and machine learning models can be trained to automatically identify defects or quality issues in products during the manufacturing process, ensuring consistent quality standards.
- Supply Chain Optimization: AI can analyze complex supply chain data to optimize inventory levels, forecast demand, and streamline logistics, reducing waste and enhancing responsiveness to market fluctuations.
- Process Automation: Robotic systems equipped with AI capabilities can perform repetitive tasks with higher accuracy and efficiency, freeing up human workers for more complex and value-added activities.
By harnessing the power of man-made intelligence manufacturers can unlock unprecedented levels of operational excellence and data-driven decision-making.
Key Benefits of Deploying AI in Production Environments
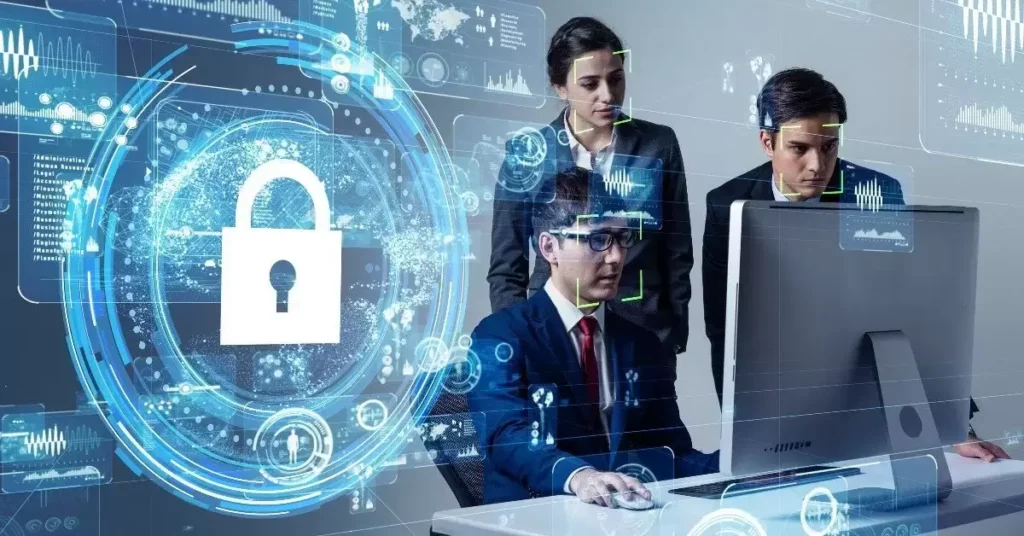
The integration of AI technologies into production networks offers a multitude of compelling benefits including:
- Increased Operational Efficiency and Reduced Downtime: Predictive maintenance capabilities enabled by AI can minimize unplanned equipment failures and reduce costly downtime leading to higher overall equipment effectiveness (OEE) and increased productivity.
- Enhanced Product Quality: Real-time monitoring and adjustments made possible by AI systems can significantly improve product quality and consistency, reducing defects, waste, and the need for rework.
- Data-Driven Insights for Process Improvement: AI algorithms can analyze vast amounts of production data, uncovering patterns and insights that would be impossible for humans to discern, leading to continuous process optimization and innovation.
- Cost Savings from Optimized Resource Allocation: AI-powered supply chain management and inventory optimization can lead to significant cost savings by ensuring the right resources are available at the right time and in the right quantities.
- Competitive Advantages in an AI-Driven Landscape: As AI becomes increasingly prevalent in manufacturing, companies that embrace man-made intelligence early will gain a crucial competitive edge over laggards positioning themselves as industry leaders.
Recommended Post: AI’s Potential: Simulated Intelligence Revolutionizing Agribusiness
Real-World Examples and Case Studies
Leading manufacturers around the sector are already figuring out the benefits of integrating man-made intelligence into their manufacturing networks. Here are some exceptional examples:
• Siemens: The German business conglomerate has applied AI-powered predictive protection solutions throughout its factories, ensuing in a mentioned 20% discount in downtime and millions in value savings.
• Intel: The chip producer has deployed laptop vision structures to look into and classify defects in their products, improving first-rate whilst reducing the want for human inspectors.
• BMW: The automaker has leveraged AI to optimize its deliver chain, leading to a stated 15-20% discount in stock fees and improved responsiveness to fluctuating purchaser call for.
• Nissan: The automotive business enterprise has correctly implemented AI-powered high-quality manipulate structures, resulting in a 70% discount in defects and a full-size improvement in universal product great.
These actual-world examples exhibit the tangible effect of synthetic intelligence on key overall performance metrics along with productiveness satisfactory and price financial savings.
Overcoming Barriers to AI Adoption in Manufacturing
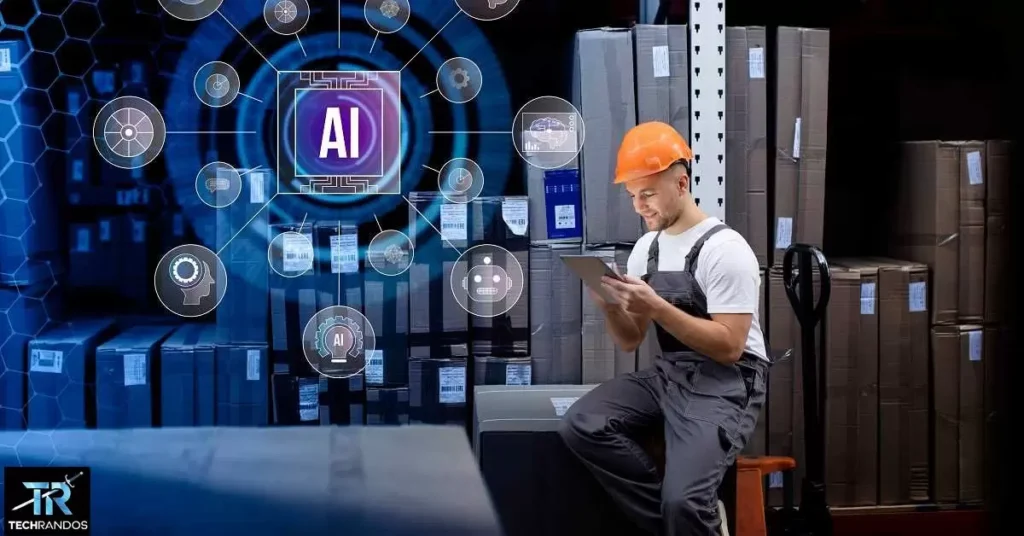
Despite the compelling benefits, the adoption of man-made intelligence in production networks is not without its challenges. Some common obstacles include:
- Data Quality and Integration Issues: AI systems heavily rely on clean, high-quality data, which can be a challenge in manufacturing environments with legacy systems and siloed data sources. Overcoming these integration hurdles is crucial for successful AI implementation.
- Workforce Reluctance and Skills Gap: The introduction of AI can sometimes face resistance from workers who fear job displacement or lack the necessary skills to work alongside intelligent systems. Addressing these concerns through transparent communication, upskilling programs, and change management strategies is essential.
- Cybersecurity and Ethical Concerns: As AI systems become more integrated into critical production processes, ensuring robust cybersecurity measures and adhering to ethical AI principles becomes paramount to mitigate risks and maintain public trust.
Strategies for addressing these challenges may include:
- Data Governance and Standardization: Implementing robust data governance frameworks and standardizing data formats to ensure high-quality, consistent data for AI systems.
- Workforce Training and Education: Investing in AI literacy programs and upskilling initiatives to equip workers with the knowledge and skills needed to thrive in an AI-driven production environment.
- Robust Cybersecurity Protocols: Adopting a “security-by-design” approach and implementing rigorous cybersecurity measures, such as access controls, encryption, and regular vulnerability assessments.
- Ethical AI Frameworks: Developing and adhering to ethical AI principles that prioritize transparency, accountability, and fairness in the development and deployment of AI systems.
By proactively addressing these challenges, organizations can pave the way for a smoother and more successful integration of man-made intelligence into their production networks.
Future Trajectory of Man-made Intelligence in Production
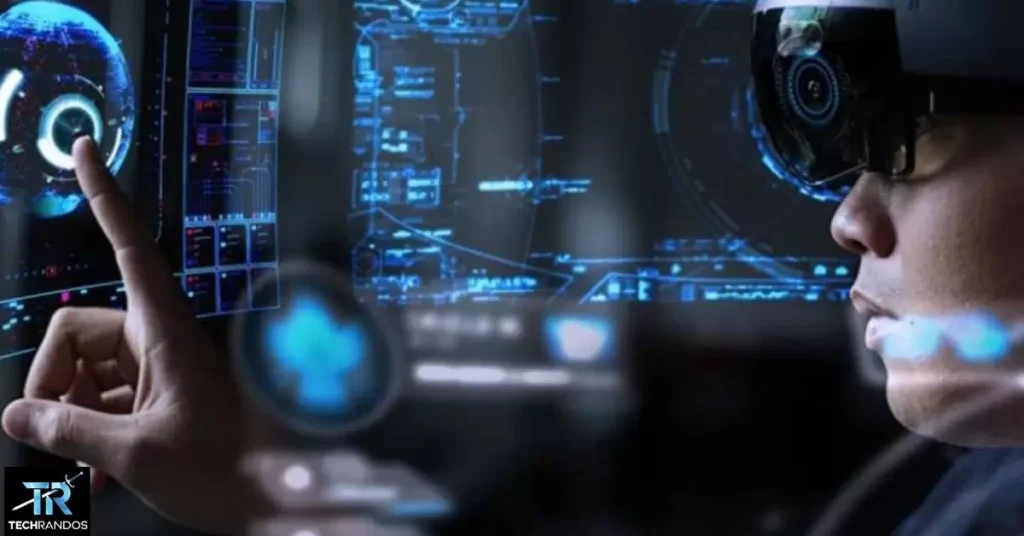
The adoption of man-made intelligence in manufacturing networks is simply the start of a transformative journey. As AI skills continue to advance we will assume even greater disruptive improvements at the horizon:
1. 5G and Edge Computing: The rollout of 5G networks and the upward push of facet computing will allow real-time information processing and decision-making closer to the source unlocking new possibilities for time-touchy packages like automated fine control and robotics.
2. Generative AI: Cutting-facet generative AI models, like the ones at the back of gear like ChatGPT, have the capacity to revolutionize product layout, ideation, and hassle-solving in manufacturing contexts.
3. Human-AI Collaboration: Rather than changing human workers, the future of AI in manufacturing will likely contain seamless collaboration among humans and clever systems, with every contributing their particular strengths for surest consequences.
To stay in advance of the curve, organizations ought to begin exploring AI implementation now and domesticate a tradition of continuous learning and innovation. By embracing guy-made intelligence, manufacturers can future-evidence their operations and role themselves as leaders in an increasingly AI-driven business panorama.
Frequently Asked Questions
What are the main applications of man-made intelligence in production networks?
The primary applications of man-made intelligence in production networks include predictive maintenance, defect detection, supply chain optimization process automation and quality control. AI-powered systems can analyze real-time data from sensors and equipment to predict potential failures identify defects in products optimize inventory levels and logistics automate repetitive tasks and ensure consistent quality standards.
How does man-made intelligence improve operational efficiency in manufacturing?
By leveraging AI technologies like machine learning and computer vision man-made intelligence can significantly improve operational efficiency in manufacturing. Predictive maintenance capabilities enabled by AI can minimize unplanned equipment downtime leading to higher overall equipment effectiveness (OEE) and increased productivity. Additionally, AI-powered process automation and optimization can streamline operations, reducing waste and enhancing resource utilization.
What are the potential cost savings from implementing man-made intelligence in production networks?
Implementing man-made intelligence in production networks can lead to significant cost savings for manufacturers. Predictive maintenance and reduced downtime can save millions in maintenance and repair costs. AI-powered supply chain optimization and inventory management can cut down on excess inventory and logistics expenses. Furthermore enhanced quality control and defect detection can reduce the need for costly rework and wastage.
How can manufacturers address workforce concerns and skills gaps when adopting AI?
To address workforce concerns and skills gaps when adopting man-made intelligence, manufacturers should implement transparent communication strategies upskilling programs and change management initiatives. Investing in AI literacy and training programs can help equip workers with the necessary knowledge and skills to work alongside intelligent systems. Additionally, fostering a culture of continuous learning and emphasizing the importance of human-AI collaboration can help alleviate fears of job displacement.
What are the cybersecurity risks associated with man-made intelligence in production networks, and how can they be mitigated?
As AI systems become more integrated into critical production processes, they can potentially introduce new cybersecurity risks. Malicious actors could attempt to compromise AI systems leading to disruptions, data breaches, or even safety hazards. To mitigate these risks manufacturers must adopt a “security-by-design” approach, implementing robust cybersecurity measures such as access controls, encryption, regular vulnerability assessments, and incident response plans.
How can manufacturers ensure the ethical and responsible deployment of man-made intelligence in production networks?
To ensure the ethical and responsible deployment of man-made intelligence in production networks manufacturers should develop and adhere to ethical AI frameworks and principles. These frameworks should prioritize transparency, accountability, fairness, and privacy in the development and deployment of AI systems. Additionally, organizations should establish governance structures and oversight mechanisms to monitor AI systems for potential biases or unintended consequences.
Conclusion
The integration of synthetic intelligence into manufacturing networks represents a paradigm shift inside the production enterprise. By harnessing the electricity of AI technology corporations can release remarkable degrees of performance best, and data-pushed insights driving innovation and aggressive blessings.
From predictive preservation and defect detection to supply chain optimization and process automation the applications of AI in manufacturing environments are extensive and swiftly evolving. As showcased by way of actual-global examples and case research early adopters of man-made intelligence are already reaping tangible advantages in phrases of decreased downtime progressed satisfactory and value savings.
However, correctly navigating the AI adventure calls for addressing challenges related to records pleasant, workforce readiness cybersecurity and ethical concerns. By imposing strong facts governance frameworks investing in team of workers upskilling adopting rigorous security protocols and adhering to moral AI ideas companies can conquer those barriers and pave the manner for a smooth and accountable integration of AI into their production networks.
As rising technologies like 5G, aspect computing, and generative AI continue to push the boundaries of what is possible the future of man-made intelligence in manufacturing networks is poised for even more disruption and innovation. Organizations that embrace this transformative shift early on will benefit a vital aggressive edge, positioning themselves as enterprise leaders in an an increasing number of AI-pushed production panorama.
The time to release the entire capacity of man-made intelligence is now. By proactively exploring AI implementation and fostering a culture of continuous studying and innovation manufacturers can future-evidence their operations and live ahead of the curve in an ever-evolving commercial landscape.