In our era of technology it has become more important, than ever to understand and evaluate visual information in various fields. Industries such as vehicles, healthcare, security and robotics are all benefiting from the advancements, in computer vision technology. This innovative field of intelligence utilizes algorithms and neural networks to enhance image analysis and object recognition to a whole new level.
The Machine Learning Foundation
Artificial intelligence, also known as AI is developed on the foundation of machine learning, a branch of AI that empowers systems to enhance their capabilities through experience, than explicit programming. In contrast, to computer vision techniques that depend on fixed rules and algorithms machine learning models can be educated using datasets enabling them to identify patterns and make informed choices based on the information they have absorbed.
An excellent illustration of this phenomenon is the application of learning networks, like Convolutional Neural Networks (CNNs) which have demonstrated great success in tasks such as categorizing images and identifying objects. Through processing training data, across layers of neurons these networks can autonomously acquire important characteristics and provide precise forecasts constantly improving their efficiency with each new instance they encounter.
Current Capabilities: Pushing the Boundaries of Visual Intelligence
The impact of simulated intelligence on computer vision is already being felt across numerous fields, enabling a wide range of applications that were previously unimaginable or highly inaccurate.
Facial Recognition
One of the most well-known applications of computer vision powered by simulated intelligence is facial recognition. By training deep learning models on massive datasets of facial images, these systems can accurately identify individuals from digital images or video frames, even accounting for variations in lighting, angle, and facial expressions.
Applications of facial recognition technology include:
- Security and Surveillance: Enhancing public safety by identifying and tracking individuals of interest.
- Access Control: Providing secure and convenient authentication for physical and digital systems.
- Retail and Advertising: Personalizing customer experiences and targeted marketing based on facial analysis.
While facial recognition technology has raised privacy concerns, ongoing research is focused on developing ethical and responsible practices to mitigate potential risks.
Object Detection and Segmentation
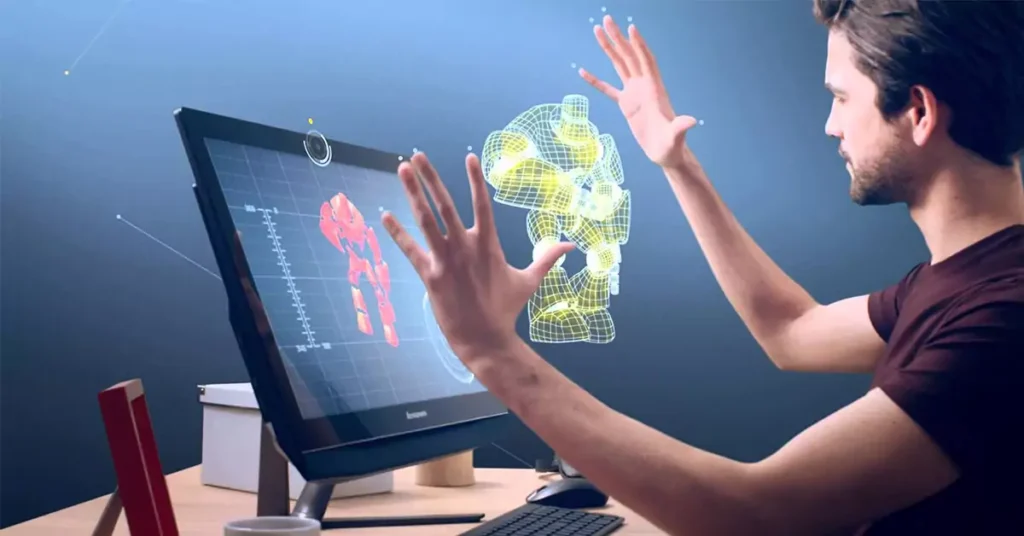
Another area where simulated intelligence has made significant strides is in object detection and segmentation. By leveraging advanced computer vision algorithms, these systems can not only locate and identify multiple objects within an image or video frame but also precisely segment and isolate individual objects, shapes, or regions of interest.
Some real-world applications of object detection and segmentation include:
- Self-Driving Cars: Accurately detecting and classifying objects on the road, such as pedestrians, vehicles, and traffic signs, is crucial for safe autonomous navigation.
- Manufacturing and Quality Control: Identifying defects, anomalies, or missing components during production processes.
- Robotics and Automation: Enabling robots to perceive and interact with their surroundings, essential for tasks like assembly, pick-and-place operations, and navigation.
- Medical Imaging: Detecting and segmenting anatomical structures, lesions, or tumors from medical scans, aiding in diagnosis and treatment planning.
By continuously improving the accuracy and speed of object detection and segmentation, simulated intelligence is paving the way for more advanced applications across various domains.
Recommended Article: Unleashing the Power of Artificial Intelligence in Healthcare
Feature Extraction and Image Analysis
Beyond object recognition, simulated intelligence is also driving significant advancements in feature extraction and image analysis. These techniques enable computers to isolate and understand essential characteristics, textures, edges, and specific shapes within visual data, extracting meaningful information that can be used for decision-making or further processing.
Examples of feature extraction and image analysis applications include:
- Medical Imaging: Identifying and analyzing patterns in medical images for disease detection, diagnosis, and treatment planning.
- Remote Sensing and Environmental Monitoring: Analyzing satellite and aerial imagery for land use, crop monitoring, and environmental impact assessments.
- Content-Based Image Retrieval: Enabling efficient search and retrieval of images based on their visual content, rather than relying on manual annotations or keywords.
- Computer Graphics and Augmented Reality: Extracting and understanding visual features to create realistic simulated environments and augmented reality experiences.
By continually refining feature extraction and image analysis capabilities, simulated intelligence is unlocking new frontiers in how we interpret and leverage visual data across various domains.
Future Possibilities: Pushing the Boundaries of Visual Intelligence
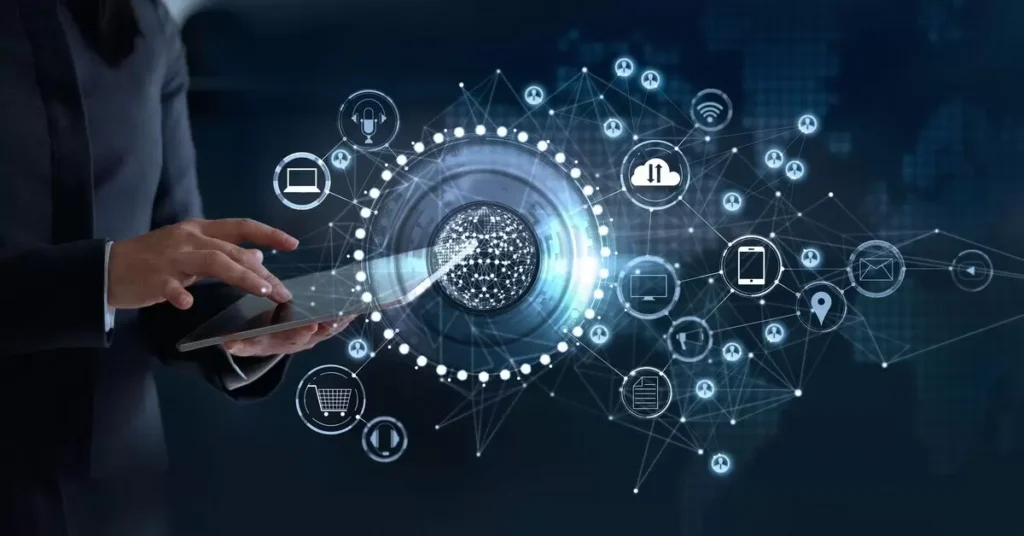
While the current applications of simulated intelligence in computer vision are already impressive, researchers and developers are constantly exploring new frontiers and pushing the boundaries of what’s possible. As computational power increases and algorithms become more sophisticated, we can expect even more transformative developments in the near future.
Real-Time Processing and Decision-Making
One area of significant interest is the ability to perform real-time processing and decision-making based on visual data. This capability is critical for applications such as autonomous driving, where split-second reactions are essential for safety, as well as security surveillance, industrial automation, and navigation systems.
By leveraging the power of simulated intelligence and specialized hardware like graphics processing units (GPUs), computer vision systems can process and analyze visual information in real-time, enabling immediate responses and decision-making based on the perceived environment.
Advancing Computer Graphics and Simulated Environments
Simulated intelligence is also poised to revolutionize the field of computer graphics and simulated environments. By combining advanced image analysis and feature extraction techniques with generative models, researchers are exploring the creation of highly realistic and dynamic virtual environments.
These simulated environments have numerous applications, including:
- Gaming and Entertainment: Creating immersive and visually stunning gaming experiences with realistic environments and character interactions.
- Architectural Visualization and Design: Enabling architects and designers to visualize and experiment with different design concepts in a simulated environment before physical construction.
- Training and Simulation: Providing safe and controlled environments for training purposes, such as pilot training, surgical simulations, or disaster response scenarios.
As the capabilities of simulated intelligence continue to expand, the line between reality and simulated environments will become increasingly blurred, opening up new realms of possibilities for interactive and immersive experiences.
Ethical Considerations and Responsible Development
While the potential benefits of simulated intelligence in computer vision are vast, it is crucial to address ethical considerations and ensure responsible development and deployment of these technologies. Issues such as privacy concerns, bias in data and algorithms, and the potential for misuse or dual-use applications must be carefully navigated.
Ongoing research and collaboration between technologists, policymakers, and ethical experts are essential to develop robust frameworks and guidelines that promote transparency, accountability, and the ethical use of simulated intelligence in computer vision applications.
Getting Started with Simulated Intelligence for Computer Vision
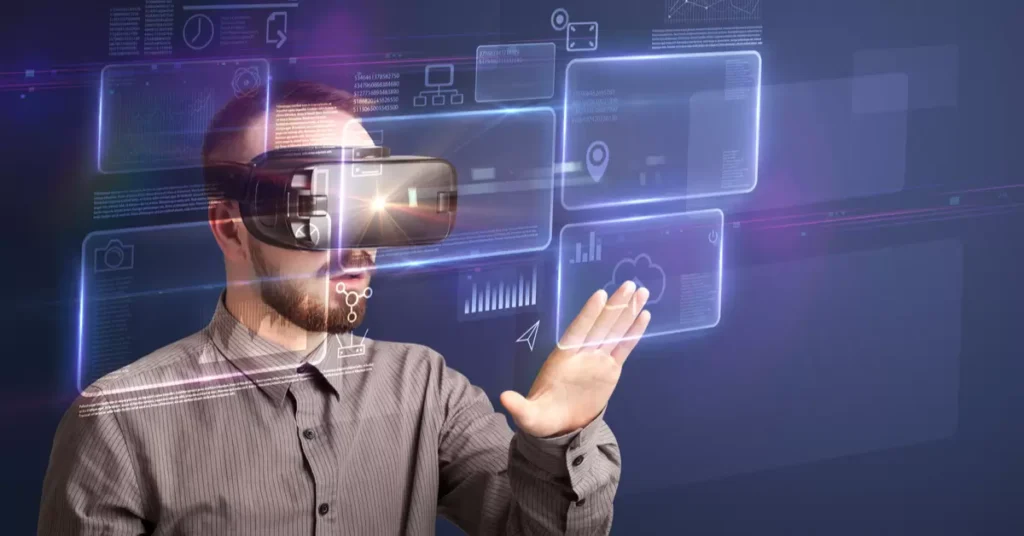
As simulated intelligence and computer vision continue to evolve and become more accessible, there are numerous opportunities for individuals and organizations to explore and leverage these powerful technologies.
One of the most exciting developments is the availability of cloud-based services and pre-trained models offered by companies like Google, Amazon, Microsoft, and others. These platforms provide developers and researchers with access to powerful computing resources and state-of-the-art machine learning models, lowering the barrier to entry and enabling rapid experimentation and prototyping.
For those looking to get started with simulated intelligence for computer vision, several beginner-friendly resources and tutorials are available online, covering topics like:
- Introduction to Machine Learning and Deep Learning: Understanding the fundamental concepts and algorithms that underlie simulated intelligence.
- Computer Vision Basics: Exploring techniques for image preprocessing, feature extraction, and object detection.
- Convolutional Neural Networks (CNNs): Hands-on experience with building and training CNNs for image classification and object detection tasks.
- Transfer Learning: Leveraging pre-trained models and fine-tuning them for specific computer vision tasks, accelerating development and improving performance.
Frequently Asked Questions
1. What is simulated intelligence, and how is it different from traditional computer vision techniques?
Simulated intelligence, also known as artificial intelligence (AI), refers to the ability of machines to simulate human-like intelligence and decision-making processes. Unlike traditional computer vision techniques that rely on hard-coded rules and algorithms, simulated intelligence leverages machine learning models that can learn and improve from experience without being explicitly programmed. This allows for more accurate and adaptive computer vision systems capable of recognizing patterns, making predictions, and continuously improving over time.
2. What are some of the current applications of simulated intelligence in computer vision?
Some current applications include facial recognition for security and authentication, object detection and segmentation for self-driving cars and robotics, feature extraction and image analysis for medical imaging and remote sensing, and content-based image retrieval. These applications are enabled by advanced techniques like deep learning neural networks, such as Convolutional Neural Networks (CNNs), which can automatically learn to extract relevant features and make accurate predictions from visual data.
3. What are the potential future developments in simulated intelligence for computer vision?
Future developments include real-time processing and decision-making for applications like autonomous driving and security surveillance, the creation of highly realistic simulated environments for gaming, training simulations, and architectural visualization, and the integration of computer vision with other emerging technologies like augmented reality and virtual reality. Additionally, researchers are exploring ways to address ethical concerns and ensure responsible development and deployment of these technologies.
4. How can individuals or organizations get started with simulated intelligence for computer vision?
One way to get started is by leveraging cloud-based services and pre-trained models offered by companies like Google, Amazon, and Microsoft. These platforms provide access to powerful computing resources and state-of-the-art machine learning models, lowering the barrier to entry for experimentation and prototyping. Additionally, there are numerous online resources and tutorials available for learning the fundamentals of machine learning, deep learning, and computer vision techniques like image preprocessing, feature extraction, and object detection.
5. What are some of the ethical considerations surrounding the use of simulated intelligence in computer vision?
Ethical considerations include privacy concerns related to facial recognition and surveillance, potential biases in the data and algorithms used to train these systems, and the potential for misuse or dual-use applications. It is crucial to develop robust frameworks and guidelines that promote transparency, accountability, and the ethical use of these technologies to mitigate potential risks and ensure responsible development and deployment.
6. How can simulated intelligence for computer vision impact industries like healthcare, manufacturing, and robotics?
In healthcare, simulated intelligence for computer vision can enable more accurate and efficient medical image analysis, disease detection, and treatment planning. In manufacturing, it can be used for quality control, defect detection, and automated assembly processes. In robotics, advanced computer vision capabilities are essential for tasks like object recognition, navigation, and manipulation, enabling robots to perceive and interact with their surroundings more effectively. Overall, these technologies have the potential to drive significant improvements in efficiency, accuracy, and automation across various industries.
Conclusion
The integration of simulated intelligence and computer vision is ushering in a new era of visual intelligence, unlocking unprecedented capabilities and transforming numerous industries. From facial recognition and object detection to advanced image analysis and decision-making, this technology is breaking down barriers and opening up new frontiers in how we perceive and interact with the world around us.
While the current applications are already impressive, the future possibilities are even more exciting, with researchers exploring real-time processing, decision-making, and the creation of highly realistic simulated environments. However, as we embrace these developments, it is crucial to address ethical considerations and ensure responsible development and deployment of these technologies.